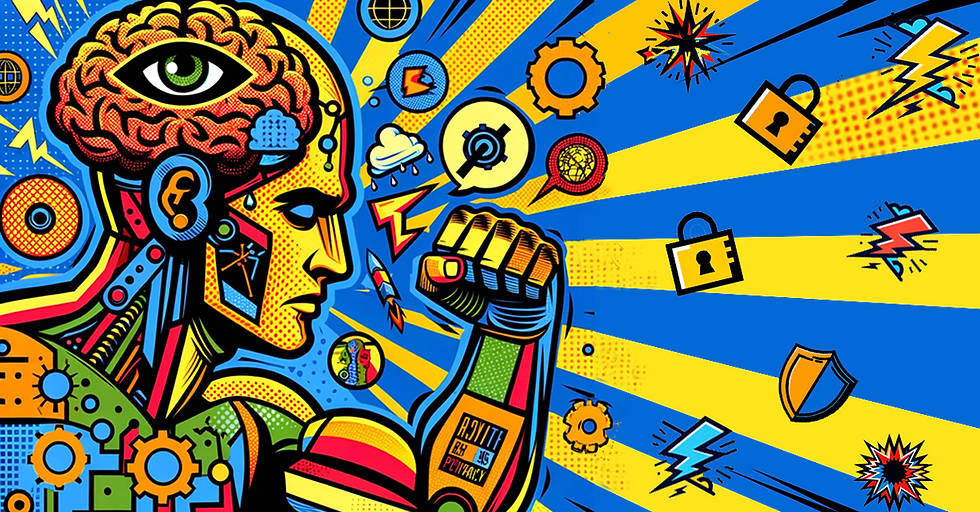
Introduction:
Artificial Intelligence (AI) is becoming a key part of our everyday lives. AI powers everything from customer service bots to sophisticated machine learning (ML) models and large language models (LLMs), the security of these systems is paramount. As AI becomes more embedded in our daily lives and corporate operations, the stakes for safeguarding these technologies from malicious exploits have never been higher.
On 21 March 2024, the European Union continued their efforts to set the global bar for the security and privacy of consumer data and trust with the passage of the first global artificial intelligence resolution. The resolution calls on member states to develop responsible AI systems to uphold human rights and comply with international law.
Problem Statement:
The rapid advancement and adoption of AI have outpaced the development of robust security measures, leaving critical systems vulnerable to a myriad of cybersecurity threats. This vulnerability not only jeopardises sensitive data but also poses significant risks to the integrity and reliability of AI-driven processes.
Current Landscape:
Frameworks and guidelines such as the OWASP Top 10 for ML and LLM, Microsoft Best Practices for AI Security Risk Management, the NIST AI Risk Management Framework, and NSCS Guidelines for securing AI system development have been established to address these concerns. Yet, the implementation remains inconsistent across industries, often due to a lack of awareness or resources.
Key AI Risks:
Data Poisoning: Malicious actors can manipulate the data used to train AI models, leading to flawed or biased outcomes. Case studies have highlighted how subtle alterations to training data can compromise the integrity of facial recognition systems, causing misidentification and bias.
Model Theft: Probing proprietary AI models allows attackers to replicate or reverse-engineer sensitive technologies. Instances of stolen ML models from tech companies have exposed intellectual property and competitive advantages to risk.
Adversarial Attacks: Attackers use manipulated inputs to trick AI systems into making erroneous decisions. Notably, adversarial examples have deceived autonomous vehicle systems into misinterpreting traffic signs, posing severe safety risks.
Impact on Industry:
AI systems' vulnerabilities can lead to significant financial losses, erosion of customer trust, and potentially catastrophic operational failures. Industries relying heavily on AI, from finance to healthcare, face unprecedented challenges in securing their AI assets against evolving threats.
Mitigation Strategies:
Robust Data Governance: Implementing strict controls over data collection, storage, and use for AI training can mitigate the risk of data poisoning.
Model Hardening: Techniques like model watermarking and encryption should be employed to protect against model theft and unauthorised use.
Adversarial Training: Incorporating adversarial examples into training strengthens AI systems against manipulation.
For your convenience:
Here is a list of key references that may be helpful in the development of your AI risk and security policy and governance documentation:
Case Studies | MITRE ATLAS™ - good for AI threat case study reference.
AI Risk Management Framework | NIST - A comprehensive AI risk management framework mapped to the security lifecycle core functions that the security community has grown to rely on.
AI Security Concerns in a Nutshell | German Federal Office of Information Security – Great technical information delivered with famous German efficiency.
Guidelines for secure AI system development | NCSC.GOV.UK – Joint publication from various nation-states on secure AI system development.
Engaging with Artificial Intelligence | Cyber.gov.au – Succinct business-friendly guideline on adopting AI.
OWASP AI Exchange | OWASP - OWASPs Top 10 threat vectors mapping and translation to ML and LLM.
Below is an aggregated list from various sources above on some questions to ask your AI stakeholders in your business:
Do you understand where accountability and responsibility for AI/ML security sit in your organisation?
Does everyone involved in ML deployment, including board members and/or senior executives, know enough about AI systems to consider their risks and benefits?
Does security factor into decisions about whether to use ML products?
How do the risks of using ML products integrate into your existing governance processes?
What are your organisation’s critical assets in terms of ML, and how are they protected?
What is the worst case (operationally or reputationally) if your organisation's ML tool fails?
How would you respond to a serious security incident involving an ML tool?
Do you understand your data, model, and ML software supply chains, and can you ask suppliers the right questions about their security?
Do you understand where your organisation may have skills or knowledge gaps related to ML security? Is a plan in place to address this?
Has your organisation implemented the cyber security frameworks relevant to its jurisdiction?
How will the system affect your organisation’s privacy and data protection obligations?
Does your organisation enforce multi-factor authentication? (FIDO2 compliant?)
How will your organisation manage privileged access to the AI system?
How will your organisation manage backups of the AI system?
Can your organisation implement a trial of the AI system?
Is the AI system secure-by-design, including its supply chain?
Does your organisation understand the limits and constraints of the AI system?
Does your organisation have suitably qualified staff to ensure the AI system is set up, maintained, and used securely?
Does your organisation conduct health checks of your AI system?
Does your organisation enforce logging and monitoring?
What will your organisation do if something goes wrong with the AI system?
Please leave a comment below if you have any more AI references or considerations that would be helpful for our fellow readership.
Conclusion:
Securing these systems cannot be overstated as AI continues to reshape industries. The journey to AI security is complex and requires a proactive and informed approach to effectively navigate the myriad of risks.
Call to Action:
We must collectively prioritise the security of AI technologies. By adopting established frameworks and guidelines, investing in continuous education, and fostering a culture of security, we can safeguard the future of AI. Let’s embark on this journey together—share your thoughts, experiences, and strategies for securing AI in your industry.
About QalatCyber Ltd
Based in the Dubai International Financial Centre Innovation Hub, QalatCyber Ltd specialises in expert cybersecurity consulting services tailored for the Middle East & Africa region's businesses. We aim to be the trusted partner organisations turn to strengthen their cyber defences amidst global digital transformation challenges.
Our services include Merger & Acquisition evaluation, Virtual CISO services, Cyber Training and Awareness programs, Executive Coaching, Cyber Assessments and Assurance, Governance and Policy development, Audit Readiness, Supplier Assessment, Project and Capability delivery support, and Higher Education Student Support.
Leveraging extensive industry experience and a dedication to excellence, QalatCyber is at the forefront of addressing the complex cybersecurity needs of today's digital landscape.
Let us help you secure your digital future today.
Contact info@qalatcyber.com with any questions about how we can help your organisation achieve its digital aspirations quickly and safely.
Kommentare